A new AI passes the smell test almost 100% of the time
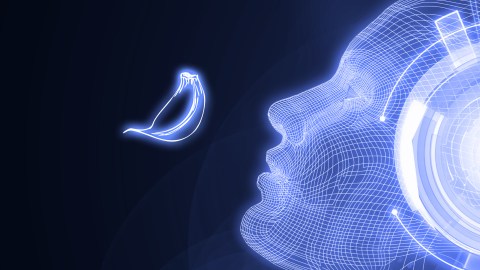
Image source: Peshkova/Ilona Rainbow/Shutterstock/Big Think
- Two researchers have created an algorithm that can accurately identify 10 different smells.
- The AI algorithm runs on an Intel chip that has 130,000 silicon "neurons."
- The natural mammalian olfactory bulb grows new neurons even in adults.
Our noses are busy beasts. At any given moment, multiple smells are competing for our attention, and somehow the brain can tell when it’s smelling an orange even against a backdrop of other scents, say perfume or soap. The brain’s olfactory bulb has hundreds of receptors tracking odors all the time, and yet somehow keeps everything straight. A scientist at Cornell University working with a researcher at Intel has just created an AI algorithm trained to recognize 10 scents by mimicking the mammalian olfactory bulb (MOB). Give the algorithm a computer chip to run on and it can learn to identify new odors.
Image source: GiroScience/Shutterstock
Simulating the brain
Thomas Cleland, senior author of “Rapid Learning and Robust Recall in a Neuromorphic Olfactory Circuit” published in Nature Machine Intelligence says, “This is a result of over a decade of studying olfactory bulb circuitry in rodents and trying to figure out essentially how it works, with an eye towards things we know animals can do that our machines can’t.”
“We now know enough to make this work,” says Cleland speaking with Cornell Chronicle. “We’ve built this computational model based on this circuitry, guided heavily by things we know about the biological systems’ connectivity and dynamics. Then we say, if this were so, this would work. And the interesting part is that it does work.”
Cleland’s co-author, Intel intern Nabil Imam, has the AI running on an Intel research chip called Loihi. The chip is something of a wonder all by itself. It’s designed to perform neuromorphic computing inspired by the brain, sporting some 130,000 silicon “neurons.” Like our own neurons, each can fire independently of the others, sending pulsed signals to its neighbors, changing their electrical states. In addition, Loihi can accept inputs from a variety of physical sensors, such as the metal-oxide chemical sensors that Cleland and Imam used, allowing the entire structure to simulate the natural learning process that occurs when the mammalian brain is fed various sensory inputs.
Image source: korkeng/VanoVasaio/Shutterstock/Big Think
What the AI knows
In the MOB, there are a couple of important neuron types: mitral cells that switch on to signify a smell has been detected but don’t identify it, and granule cells that learn to become specialized and pinpoint chemicals in the smell. This is the process Cleland and Imam have programmed Loihi to imitate when odors are presented to the system’s sensors.
The researchers have trained the algorithm to identify 10 chemical smells: acetone, acetaldehyde, ammonia, butanol, ethylene, methane, methanol, carbon monoxide, benzene, and toluene. The idea is that in the presence of an odor, Loihi verifies the scent’s presence and patterns of firing neurons identify exactly which smell it is.
Over the course of five cycles of exposure to each odor, the AI was eventually able to associate specific neural firing patterns with specific smells. The AI correctly identified eight of the smells 100 percent of the time, and the remaining two with 90 percent accuracy.
Additional simulations were run in which the target odor was masked 80 percent by other smells, the way they’d often be in the real word. In these tests, accuracy dropped down to just 30 percent, though that’s still pretty impressive for such a “young” AI. Says Cleland, “The pattern of the signal has been substantially destroyed, and yet the system is able to recover it.”
Image source: Patrick J. Lynch, wikimedia
The sweet smell of success
As AI and machine learning do, Cleland’s and Imam’s algorithm would be expected to get better with more training. Machine smell-detection for use in bomb-sniffing, for example, is clearly not just around the corner. Still, the research offers new insights about learning.
Due to the normally finite number of neurons available for storage of new memories in an adult brain, and the fact that “when you learn something, it permanently differentiates neurons,” as Cleland says, storing new information means changing the information already encoded in neurons. However, the mammalian olfactory bulb is able to continually learn smells — hundreds or thousands of them —without losing knowledge of already-learned odors. It’s one of the few areas of the brain that can keep creating new neurons through adulthood.
The researchers’ experience with Loihi underscores the value of the olfactory bulb’s unusual neuronal expandability. “The computational model turns into a biological hypothesis for why adult neurogenesis is important,” Cleland says, “because it does this thing that otherwise would make the system not work. So in that sense, the model is feeding back into biology. And in this other sense, it’s the basis for a set of devices for artificial olfactory systems that can be constructed commercially.”