Will AI replace mathematicians?
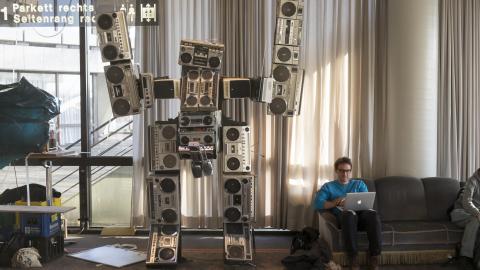
Credit: Patrick Lux via Getty Images
- Most everyone fears that they will be replaced by robots or AI someday.
- A field like mathematics, which is governed solely by rules that computers thrive on, seems to be ripe for a robot revolution.
- AI may not replace mathematicians but will instead help us ask better questions.
The following is an excerpt adapted from the book Shape. It is reprinted with permission of the author.
Will machines replace us? Since the origin of artificial intelligence (AI), people have worried that computers eventually (or even imminently!) will surpass the human cognitive capacity in every respect.
Artificial intelligence pioneer Oliver Selfridge, in a television interview from the early 1960s, said, “I am convinced that machines can and will think in our lifetime” — though with the proviso, “I don’t think my daughter will ever marry a computer.” (Apparently, there is no technical advance so abstract that people can’t feel sexual anxiety about it.)
AI Anxiety
Let’s make the relevant question more personal: will machines replace me? I’m a mathematician; my profession is often seen from the outside as a very complicated but ultimately purely mechanical game played with fixed rules, like checkers, chess, or Go. These are activities in which machines have already demonstrated superhuman ability.
Some people imagine a world where computers give us all the answers. I dream bigger. I want them to ask good questions.
But for me, math is different: it is a creative pursuit that calls on our intuition as much as our ability to compute. (To be fair, chess players probably feel the same way.) Henri Poincaré, the mathematician who re-envisioned the whole subject of geometry at the beginning of the 20th century, insisted it would be hopeless
“to attempt to replace the mathematician’s free initiative by a mechanical process of any kind. In order to obtain a result having any real value, it is not enough to grind out calculations, or to have a machine for putting things in order: it is not order only, but unexpected order, that has a value. A machine can take hold of the bare fact, but the soul of the fact will always escape it.”
But machines can make deep changes in mathematical practice without shouldering humans aside. Peter Scholze, winner of a 2018 Fields Medal (sometimes called the “Nobel Prize of math”) is deeply involved in an ambitious program at the frontiers of algebra and geometry called “condensed mathematics” — and no, there is no chance that I’m going to try to explain what that is in this space.
Meet AI, your new research assistant
What I am going to tell you is the result of what Scholze called the “Liquid Tensor Experiment.” A community called Lean, started by Leonardo de Moura of Microsoft Research and now open-source and worldwide, has the ambitious goal of developing a computer language with the expressive capacity to capture the entirety of contemporary mathematics. A proposed proof of a new theorem, formalized by translation into this language, could be checked for correctness automatically, rather than staking its reputation on fallible human referees.
Scholze asked last December whether the ideas of condensed mathematics could be formalized in this way. He also wanted to know whether it could express the ideas of a particularly knotty proof that was crucial to the project — a proof that he was prettysure was right.
When I first heard about Lean, I thought it would probably work well for some easy problems and theorems. I underestimated it. So did Scholze. In a May 2021 blog post, he writes, “[T]he Experiment has verified the entire part of the argument that I was unsure about. I find it absolutely insane that interactive proof assistants are now at the level that within a very reasonable time span they can formally verify difficult original research.”
And the contribution of the machine wasn’t just to certify that Scholze was right to think his proof was sound; he reports that the work of putting the proof in a form that a machine could read improved his own human understanding of the argument!
The Liquid Tensor Experiment points to a future where machines, rather than replacing human mathematicians, become our indispensable partners. Whether or not they can take hold of the soul of the fact, they can extend our grasp as we reach for the soul.
Slicing up a knotty problem
That can take the form of “proof assistance,” as it did for Scholze, or it can go deeper. In 2018, Lisa Piccirillo, then a PhD student at the University of Texas, solved a long-standing geometry problem about a shape called the Conway knot. She proved the knot was “non-slice” — this is a fact about what the knot looks like from the perspective of four-dimensional beings. (Did you get that? Probably not, but it doesn’t matter.) The point is this was a famously difficult problem.
A few years before Piccirillo’s breakthrough, a topologist named Mark Hughes at Brigham Young had tried to get a neural network to make good guesses about which knots were slice. He gave it a long list of knots where the answer was known, just as an image-processing neural net would be given a long list of pictures of cats and pictures of non-cats.
Hughes’s neural net learned to assign a number to every knot; if the knot were slice, the number was supposed to be 0, while if the knot were non-slice, the net was supposed to return a whole number bigger than 0. In fact, the neural net predicted a value very close to 1 — that is, it predicted the knot was non-slice — for every one of the knots Hughes tested, except for one. That was the Conway knot.
For the Conway knot, Hughes’s neural net returned a number very close to 1/2, its way of saying that it was deeply unsure whether to answer 0 or 1. This is fascinating! The neural net correctly identified the knot that posed a really hard and mathematically rich problem (in this case, reproducing an intuition that topologists already had).
Some people imagine a world where computers give us all the answers. I dream bigger. I want them to ask good questions.
Dr. Jordan Ellenberg is a professor of mathematics at the University of Wisconsin and a number theorist whose popular articles about mathematics have appeared in the New York Times, the Wall Street Journal, Wired, and Slate. His most recent book is Shape: The Hidden Geometry of Information, Biology, Strategy, Democracy, and Everything Else.