AI Can See America’s Wealth Inequality From Space
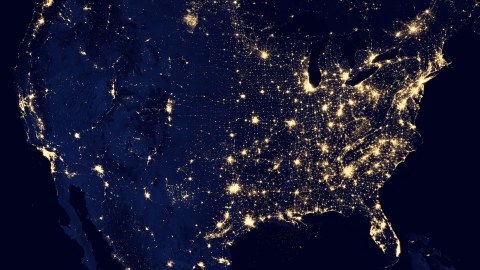
What does wealth look like… from space? This is the question researchers from Carnegie Mellon University wanted to answer when they built Penny. Penny is an AI built on machine learning using neural networks. Through an interactive website Penny lets you browse satellite images of New York City and St. Louis and see how different kinds of features make an area look wealthy or poor to the AI.
To build Penny, the researchers used household income data from the U.S. Census Bureau, mapped it, and overlaid it with satellite imagery. They color-coded areas to represent different income brackets. In the picture below, green represents areas with the highest quartile of annual income (averaging $71,876 and above), red represents areas with the lowest annual income (averaging $34,176 and below) and orange and yellow represent the middle levels of income (averaging between $34,176 and $49,904, and $49,904 and $71,876, respectively).
Census data mapped and color coded / penny.digitalglobe.com
The two layers of data — the color-coded map and the satellite images — were then given to a neural network which was trained to predict the average household incomes in any area in the city. The AI looks for patterns in the imagery that correlate with census data. Over time, the neural network learns what patterns best predict high and low income levels. The model can then be used to predict income levels for a place, just by looking at a satellite image.
So, what do wealth and poverty look like from space? Some of the correlations that Penny found were that lower income areas, for example, tend to have baseball diamonds, parking lots, and large similarly shaped buildings (such as housing projects). In middle income areas there were more single-family homes and apartment buildings. Higher income areas tend to have greener spaces, tall shiny buildings, and single-family homes with lush backyards.
Patterns of low-income areas / penny.digitalglobe.com
Patterns of high-income areas / penny.digitalglobe.com
There has also been other research concerned with estimating wealth from satellite data. Scientists from the University of Konstanz found thatit is possible to make inferences about the wealth of a region, as well as individual settlements, by measuring the amount of nighttime illumination.
An interesting feature of Penny is that the tool lets you play around with the landscape of the city, by adding and removing urban features like buildings, parks, and freeways. After making the changes, Penny makes a new estimate on the income bracket of the block.
Penny’s interface allows the users to drag and drop urban features on the map. / penny.digitalglobe.com
The researchers are hoping to spark a conversation about artificial intelligence, machine learning, cities, infrastructure, satellite imagery, and big data. The way machines understand these things has increasingly important implications for how we understand patterns of urbanization, wealth, and the human condition.