Young children trounce large language models in a simple problem-solving task
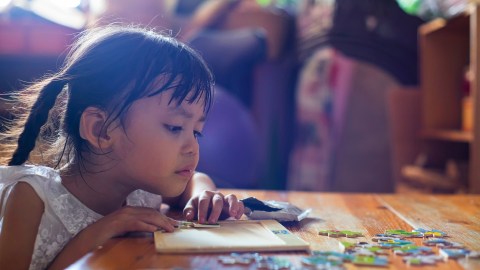
- Despite their genuine potential to change how society works and functions, large language models get trounced by young children in basic problem-solving tasks testing their ability to innovate, according to new research.
- The study reveals a key weakness of large language models: They do not innovate.
- If large language models can someday become innovation engines, their programmers should try to emulate how children learn, the authors contend.
Large language models like ChatGPT are immensely powerful — the first artificial intelligences to produce truly human-like content. They are already being used to provide customer service, write software, and aid in drug discovery, among many other applications. But despite their genuine potential to change how society works and functions, large language models get trounced by young children in basic problem-solving tasks testing the ability to innovate, according to new research.
Doctoral students Eunice Yiu and Eliza Kosoy and their laboratory lead Dr. Alison Gopnik recently detailed the findings in the journal Perspectives on Psychological Science. The trio of developmental psychologists at the University of Berkeley investigated exactly how children acquire “sophisticated understandings and representations of the causal world around them.”
In their experiment, they challenged children ages three to seven with various problems testing their ability to innovate with tools. Kids were presented with a variety of situations where they were asked to complete a task without the proper tool: drawing a circle without a compass, for example. They were then asked to choose one of three other objects to complete the task at hand: one that was associated with, but not functionally relevant to, the context; one that was superficially dissimilar but had the same causal properties as the tool needed; and a totally irrelevant object.
In the case of trying to draw a circle, kids were asked to choose between a ruler, a teapot, and a stove. The teapot is the correct answer because one can simply trace its base to create a circle. Young children were very good at sussing out the correct answer in these tests, getting them correct about 85% of the time.
But when Yiu, Kosoy, and Gopnik presented the same problems in written form to numerous large language models including ChatGPT, the AIs faltered, unable to match the children’s success. GPT-4 came the closest with 76% correct. None of the others, including OpenAI’s GPT-3.5 Turbo, Anthropic’s Claude, and Google’s FLAN-T5 were in the ballpark, often opting for a ruler to draw a circle.
The authors speculated on large language models’ lackluster performance.
“Discovering novel functions in everyday tools is not about finding the statistically nearest neighbor from lexical co-occurrence patterns. Rather, it is about appreciating the more abstract functional analogies and causal relationships between objects that do not necessarily belong to the same category or are associated in text. In these examples, people must use broader causal knowledge, such as understanding that tracing an object will produce a pattern that matches the object’s shape to produce a novel action that has not been observed or described before.”
Imitation versus innovation
The experiment reveals a key weakness of large language models: While they are remarkable imitation engines, repurposing and spreading what’s already known and created, they do not introduce novel ideas. To illustrate, if large language models existed in a world where the only things that could fly were birds, and you asked one to devise a flying machine, they would never come up with an airplane.
“The best way to think of these systems is as powerful new cultural technologies, analogous to earlier technologies such as writing, print, libraries, the Internet, and even language itself,” the authors wrote.
Could large language models ever become innovation engines? If so, their programmers should try to emulate how children learn, Yiu, Kosoy, and Gopnik contend.
“What [AI] systems would need is some kind of embodied active, curious intervention and experimentation on the real world,” Gopnik told Big Think in an email interview. “There are some beginnings of this in robotics, but it is still far away.”
Gopnik and her co-authors provided additional detail in the paper.
“Although we do not know the details of children’s learning algorithms or data, we do know that, unlike large language and language-and-vision models, children are curious, active, self-supervised, and intrinsically motivated. They are capable of extracting novel and abstract structures from the envi- ronment beyond statistical patterns.”
“Babies seem to learn much more general and powerful kinds of knowledge than AIs do, from much less and much messier data. In fact, human babies are the best learners in the universe,” Gopnik wrote in a 2019 op-ed in the Wall Street Journal.
It’s a wonderful irony: When AIs become more childlike, that’s when their abilities might truly explode, with world-changing ramifications.