Predicting the president: Two ways election forecasts are misunderstood
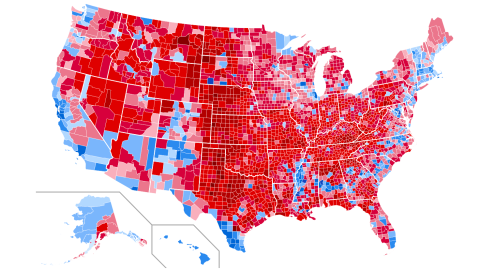
- There are two common misconceptions that muddy people’s understanding of election forecasting, says Eric Siegel: Blaming the prognosticator and predicting candidates versus predicting voters.
- In 2016, Nate Silver’s forecast put about 70% odds on Clinton winning. Despite people’s shock at the election results, that forecast was not wrong.
- As predictions for the 2020 presidential election ramp up, it’s important to understand what election forecasting means and to bust the misconceptions that warp our expectations.
When it’s a presidential election year, speculation is in the cards. It’s the national pastime. Everyone wants to predict who’ll win.
But, man, did people mismanage their own expectations leading up to the 2016 presidential election, when Donald Trump defeated Hillary Clinton.
This was due in no small part to the misinterpretation of election forecasts. There are two common misconceptions, and correcting them comes down to the fundamental idea of what a probability is.
In 2016, Nate Silver’s forecast put about 70% odds on Clinton winning. Who’s Nate? There’s no more better known person of prediction in this country, no more famous prognostic quant than former New York Times blogger and political poll aggregator Nate Silver, who had gained notoriety for correctly predicting the outcome of the 2012 presidential election for each individual state.
Presently, his up-to-the-minute forecast of the 2020 Democratic Primary is live, and his forecast of the 2020 general election is forthcoming.
By the way, number crunching serves more than just to forecast presidential elections – it also helps win presidential elections. Click here to read all about it.
Nate Silver speaks at a panel in New York City.
Photo: Krista Kennell/Patrick McMullan via Getty Images
Misconception #1: Blaming the prognosticator
When Clinton lost in 2016, everyone was like, “OMG, epic fail!” The reasoning was, well, the 70% forecast that she would win had proven to be wrong, so the problem must have been either bad polling data or something about Silver’s model, or both.
But no – the forecast wasn’t bad! “70%” does not mean Clinton will clearly win. And a 30% chance of Trump winning isn’t a long shot at all. Something that happens 30% of the time is really pretty common and normal. And that’s what a probability is. It means that, in a situation just like this, it will happen 30 out of 100 times, that is, 3 out of 10 times. Those aren’t long odds.
And Clinton’s 70% probability is actually closer to a 50/50 toss-up than it is to a 100% “sure thing.” When you see “70%,” the take-away isn’t that Clinton is pretty much a shoe-in. No, the take-away is, “I dunno.” Lot’s of uncertainty.
I believe many people saw that “70%,” and the thought process was like, “70% is a passing grade, so Clinton will definitely pass, so Clinton will definitely win.”
Prediction is hard. To be more specific, there are many situations where the outcome is uncertain and we just can’t be confident about what to expect. Nate Silver’s model looked at the data and said this one was one of those situations. Now, a confident prediction may feel more satisfying. We all want definitive answers. But it’s better for you to shrug your shoulders than to express confidence without a firm basis to do so, and it’s better for the math to do the same thing.
Press the press to give it a rest
So, I feel kind of bad for Nate Silver. He totally got a bad rap. Most of the other prominent models at large actually put Clinton’s chances much higher – between 92% and 99%. Those models exhibited overconfidence. Silver’s model didn’t strongly commit. It expressed, first and foremost, uncertainty.
Even the Harvard Gazette, in an article that ultimately defended Silver, put it this way: “Even leading statistical analysis site FiveThirtyEight.com [that’s Silver’s site] gave Donald Trump a less than 1 in 3 chance of winning. So when he surged to victory… stunned political pundits blamed pollsters and forecasters, proclaiming ‘the death of data.'”
It’s like the journalist couldn’t wrap her head around the fact that “less 1 in 3” – specifically a 30% chance – isn’t remote odds. If there were a 30% chance a car would crash, you obviously wouldn’t get in the car.
Nate Silver wasn’t betting his life on one candidate or the other. His job as a forecaster wasn’t to magically predict like a crystal ball. It was to tell you the odds as precisely as possible.
When asked by the same journalist whether he was saying he diverged from the general sentiment that polling had been a “massive failure,” Silver said, “Not only am I not on that bandwagon, I think it’s pretty irresponsible when people in the mainstream media perpetuate that narrative… We think our general election model was really good. It said there was a pretty good chance of Trump winning… if everyone says ‘Trump has no chance’ and you use modeling to say ‘Hey, look at this more rigorously; he actually has a pretty good chance. Not 50 percent, but 30 percent is pretty good.’ To me, that’s a highly successful application of modeling.”
I even remember hearing him have to talk down his coworkers on his own podcast just before the election, who were talking about Clinton’s election as a done deal. It’s like nobody understands what “30%” means.
Forecasting isn’t futurism
When you’re a contestant on the TV quiz show Jeopardy, you only buzz in when you think you know the answer to the question, cause if you get it wrong, you get penalized. So you gauge your own confidence, your own certainty that the answer you have will turn out to be correct. IBM’s Watson computer that competed against human champions on that TV show did exactly that. Its predictive model served not only to select the answer to a question, it also provided a gauge of confidence in that answer, which directly informed whether or not the computer buzzed in to answer the question at all.
Here’s my big prediction: Futurism will be entirely out of style within 20 years. Ha-ha – get it? My point is, forecasts aren’t like futurism. Futurism is the practice of putting your entire reputation down on one confident bet. In contrast, forecasting judiciously allows for uncertainty – it even calls for it, as needed.
Hillary Clinton and Donald Trump at the first presidential debate of the 2016 presidential election at Hofstra University
Photo: Getty Images
Misconception #2: Predicting candidates versus predicting voters
The other common election forecast misconception is that the “70%” estimated how much of the votes Clinton would get. That’s very much not the same thing as the chances of winning. Poll aggregators like Silver forecast which candidate will win; any forecast they also make about the percent of voters is secondary and distinct from the main probabilistic forecast.
After all, presidential races are much closer than 70/30. 2016 came out at 46% Trump against 48% Clinton, nationwide.
Now, if the data had us expecting one candidate would actually get 70% of the votes nationwide, then the chances of them winning would indeed be close to a sure thing – and a landslide victory at that. In that case, maybe they would actually end up getting less, like 60% – but that’s still a likely electoral college win. And the chances are particularly slim that the outcome would land even further away from the expected 70%, down to below 50%, so a loss of the election would be a long shot, perhaps only a 1% chance. So, if you’ve forecasted a candidate will get 70% of the votes, that may translate to more like a 99% probability of winning.
Transforming polls to probabilities
Anyway, the 70% wasn’t the expected proportion of votes. The expected proportion of votes is the input to Nate Silver’s model not the output. To be more precise, the model inputs polls, which estimate how many will vote for each candidate, and outputs a forecast, the probability that a given candidate will win.
An election poll does not constitute magical prognostic technology – it is plainly the act of voters explicitly telling you what they’re going to do. It’s a mini-election dry run.
But there’s a craft to aggregating polls, as Silver has mastered so adeptly. His model cleverly weighs large numbers of poll results, based on how many days or weeks old the poll is, the track record of the pollster, and other factors.
So Silver’s model turns poll results into a forecasted probability. It maps from one to the other. That’s what a predictive model does in general. It takes the data you have as input, and formulaically transforms it to a probability of the outcome or behavior you’re seeking to foresee.
Often, model probabilities come closer to 50% than 100%. They’re uncertain, like when your Magic Eight Ball says, “The outlook is hazy.” It can be hard to sit with and accept a lack of certainty. When the stakes are high, we’d prefer to feel confident, to know how it’s going to turn out. Don’t let that impulse draw you to a false narrative. Practice not knowing. Shrug your shoulders more. It’s good for you.
– – –
Eric Siegel, Ph.D., founder of the Predictive Analytics World and Deep Learning World conference series and executive editor of The Machine Learning Times, makes the how and why of predictive analytics (aka machine learning) understandable and captivating. He is the author of the award-winning book Predictive Analytics: The Power to Predict Who Will Click, Buy, Lie, or Die, the host of The Dr. Data Show web series, a former Columbia University professor, and a renowned speaker, educator, and leader in the field. Follow him at @predictanalytic.