The statistical significance scandal: The standard error of science?
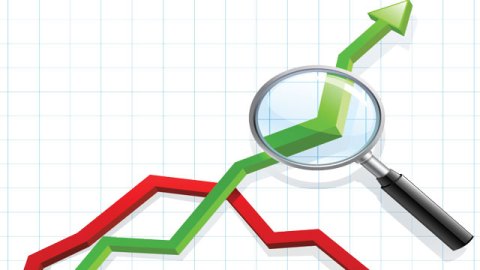
Pmis)understood as indicating that the findings have a one in twenty chance of being incorrect. The phenomenon has become a somewhat universal barrier which scientists must cross but in many cases has also inadvertently become a barrier to readers of scientific research accessing the very important numbers often hidden underneath this indication of statistical significance. The US Supreme Court for example has investigated cases where statistical significance of findings in medical trials has been used in place of undisclosed adverse events:
“In a nutshell, an ethical dilemma exists
when the entity conducting the significance test
has a vested interest in the outcome of the test.”
In a paper by the same authors written in plain English titled The Cult of Statistical Significance, a fantastic analogy is given of a hypothetical pill that would be determined useless based on a measure of statistical significance and a pill that would be determined as of statistically significant value despite being patently useless in real terms. We then hear of a real case study concerning Merck’s Vioxx painkiller marketed in over eighty countries with a peak value of over two and a half billion. After a patient died of a heart attack it emerged in court proceedings that Merck had allegedly omitted from their research findings published in the Annals of Internal Medicine that five of the patients who participated in the clinical trial of Vioxx suffered heart attacks while participating in the trial while only one participant had a heart attack while taking the generic alternative naproxen. Most worryingly of all, this was technically a correct action to take due to the fact that the Annals of Internal Medicine has strict rules regarding statistical significance of findings:
“The signal-to-noise ratio did not rise to 1.96, the 5% level of significance that the Annals of Internal Medicine uses as strict line of demarcation, discriminating the “significant” from the insignificant, the scientific from the non-scientific… Therefore, Merck claimed, there was no difference in the effects of the two pills. No difference in oomph, they said, despite a Vioxx disadvantage of about 5-to-1.”
Only after the families of dead clinical trial participants brought the matter to attention did it emerge that:
“eight in fact [of the trial participants] suffered or died in the clinical trial, not five. It appears that the scientists, or the Merck employees who wrote the report, simply dropped the three observations.”
Weirdly, the number of heart attacks that were mysteriously not reported is the very number of heart attacks required to result in the five heart attacks having no statistical significance and therefore no right impacting the outcome reported in the Annals of Internal Medicine. The paper concludes with a resounding echo from the conclusion of a paper published in American Statistician 1975:
“Small wonder that students have trouble [learning significance testing]. They may be trying to think.”
The problem of scientists manipulating data in order to achieve statistical significance, labelled p-hacking is incredibly hard to track down due to the fact that the data behind statistical significance is often unavailable for analysis by anyone other than those who did the research and themselves analysed the data.
This is where things get a bit meta. A recently developed method for identifying p-hacking involves analysing factors used to measure the significance levels of various trials and testing to see if findings of significance are significantly likely to occur excessively near to the entry level barrier required to achieve statistical significance. If this is the case, the raw unpublished data is requested and the data points in the study are assessed for patterns that indicate p-hacking. Uri Simonsohn, the researcher developing this method has already applied the technique to catch Dirk Smeesters, who has since resigned after an investigation found he massaged data to produce positive outcomes in his research. The paper has now been retracted with the note:
“Smeesters also disclosed that he had removed data related to this article in order to achieve a significant outcome”
Simonsohn has since tested his method using data collected from Diederik Stapel, the Dutch researcher who allegedly fabricated data in over thirty publications, an allegation that rocked the scientific community earlier this year. Simonsohn has not stopped there and according to an interview published in Nature earlier this year and a pre-print of a paper by Simonsohn that is now available, Simonsohn is continuing to uncover cases of research fraud using statistical techniques.
Joe Simmons and Uri Simonsohn, the researchers who devised the method, have proposed three simple pieces of information that scientists should include in an academic paper to indicate that the data has not been p-hacked. In what must certainly take the award for the most boldly humorous addition to an academic paper I have ever seen, the researchers have suggested their three rules can be remembered with a song, sung to a well known tune:
If you are not p-hacking and you know it, clap your hands.
If you determined sample size in advance, say it.
If you did not drop any variables, say it.
If you did not drop any conditions, say it.
Choir: There is no need to wait for everyone to catch-up with your desire for a more transparent science. If you did not p-hack a finding, say it, and your results will be evaluated with the greater confidence they deserve.
Why not give the song a go yourself to the tune below and firmly cement the rules in your memory (and the memories of those lucky souls that happen to currently be in your immediate vicinity).
Just in case this wasn’t quite the poignant ending to this article you were expecting, please allow me to leave you with a more dignified conclusion, courtesy of Princeton/Yale mathematician Charles Seife, taken from his tremendous lecture earlier this year which you can view below:
“Statistical significance is responsible for more idiotic ideas in the scientific literature than anything else” – Charles Seife
References:
Goodman S. (2008) A dirty dozen: twelve p-value misconceptions. Seminars in hematology, 45(3), 135-40. PMID: 18582619 Available online at: http://xa.yimg.com/kq/groups/18751725/636586767/name/twelve+P+value+misconceptions.pdf
Simmons, J. Nelson, L. and Simonsohn, U. (2012) A 21 Word Solution. Dialogue: The Official Newsletter of the Society for Personality and Social Psychology. Volume 26, No.2, Fall, 2012. : http://www.spsp.org/resource/resmgr/dialogue/dialogue_26(2).pdf
Simonsohn, Uri, Just Post It: The Lesson from Two Cases of Fabricated Data Detected by Statistics Alone (November 21, 2012). Available at SSRN: http://ssrn.com/abstract=2114571 or http://dx.doi.org/10.2139/ssrn.2114571
Yong, E. (2012) The data detective. Nature Magazine. Available online at: http://www.nature.com/news/the-data-detective-1.10937
Ziliak, S. McCloskey, D. (2012) MATRIXX INITIATIVES, INC., ET AL., Petitioners,v. JAMES SIRACUSANO AND NECA-IBEW PENSION FUND, Respondents.BRIEF OF AMICI CURIAE STATISTICS EXPERTS PROFESSORS DEIRDRE N. McCLOSKEY AND STEPHEN T. ZILIAK IN SUPPORT OF RESPONDENTS. No. 09-1156 Available at:http://www.americanbar.org/content/dam/aba/publishing/preview/publiced_preview_briefs_pdfs_09_10_09_1156_RespondentAmCu2Profs.authcheckdam.pdf
Ziliak, S. McCloskey, D. (2009) The Cult of Statistical Significance. Section on Statistical Education – JSM. Available online at: http://www.deirdremccloskey.com/docs/jsm.pdf