The consciousness battle
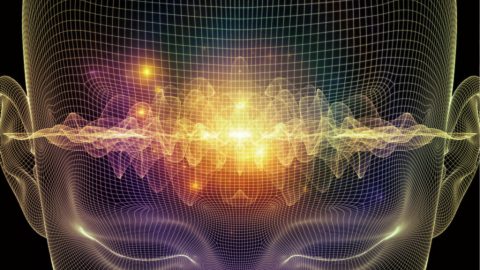
Imagine you’re at a magic show, in which the performer suddenly vanishes. Of course, you ultimately know that the person is probably just hiding somewhere. Yet it continues to lookas if the person has disappeared. We can’t reason away that appearance, no matter what logic dictates. Why are our conscious experiences so stubborn?
The fact that our perception of the world appears to be so intransigent, however much we might reflect on it, tells us something unique about how our brains are wired. Compare the magician scenario with how we usually process information. Say you have five friends who tell you it’s raining outside, and one weather website indicating that it isn’t. You’d probably just consider the website to be wrong and write it off. But when it comes to conscious perception, there seems to be something strangely persistent about what we see, hear and feel. Even when a perceptual experience is clearly ‘wrong’, we can’t just mute it.
Why is that so? Recent advances in artificial intelligence (AI) shed new light on this puzzle. In computer science, we know that neural networks for pattern-recognition—so-called deep learning models—can benefit from a process known as predictive coding. Instead of just taking in information passively, from the bottom up, networks can make top-down hypotheses about the world, to be tested against observations. They generally work better this way. When a neural network identifies a cat, for example, it first develops a model that allows it to predict or imagine what a cat looks like. It can then examine any incoming data that arrives to see whether or not it fits that expectation.
The trouble is, while these generative models can be super efficient once they’re up and running, they usually demand huge amounts of time and information to train. One solution is to use generative adversarial networks (GANs)—hailed as the ‘coolest idea in deep learning in the last 20 years’ by Facebook’s head of AI research Yann LeCun. In GANs, we might train one network (the generator) to create pictures of cats, mimicking real cats as closely as it can. And we train another network (the discriminator) to distinguish between the manufactured cat images and the real ones. We can then pit the two networks against each other, such that the discriminator is rewarded for catching fakes, while the generator is rewarded for getting away with them. When they are set up to compete, the networks grow together in prowess, not unlike an arch art-forger trying to outwit an art expert. This makes learning very efficient for each of them.
GANS and the brain
As well as a handy engineering trick, GANs are a potentially useful analogy for understanding the human brain. In mammalian brains, the neurons responsible for encoding perceptual information serve multiple purposes. For example, the neurons that fire when you see a cat also fire when you imagine or remember a cat; they can also activate more or less at random. So whenever there’s activity in our neural circuitry, the brain needs to be able to figure out the cause of the signals, whether internal or external.
We can call this exercise perceptual reality monitoring. John Locke, the 17th-century British philosopher, believed that we had some sort of inner organ that performed the job of sensory self-monitoring. But critics of Locke wondered why Mother Nature would take the trouble to grow a whole separate organ, on top of a system that’s already set up to detect the world via the senses. You have to be able to smell something before you can go about deciding whether or not the perception is real or fake; so why not just build in a check to the detecting mechanism itself?
In light of what we now know about GANs, though, Locke’s idea makes a certain amount of sense. Because our perceptual system takes up neural resources, parts of it get recycled for different uses. So imagining a cat draws on the same neuronal patterns as actually seeing one. But this overlap muddies the water regarding the meaning of the signals. Therefore, for the recycling scheme to work well, we need a discriminator to decide when we are seeing something versus when we’re merely thinking about it. This GAN-like inner sense organ—or something like it—needs to be there to act as an adversarial rival, to stimulate the growth of a well-honed predictive coding mechanism.
If this account is right, it’s fair to say that conscious experience is probably akin to a kind of logical inference. That is, if the perceptual signal from the generator says there is a cat, and the discriminator decides that this signal truthfully reflects the state of the world right now, we naturally see a cat. The same goes for raw feelings: pain can feel sharp, even when we know full well that nothing is poking at us, and patients can report feeling pain in limbs that have already been amputated. To the extent that the discriminator gets things right most of the time, we tend to trust it. No wonder that when there’s a conflict between subjective impressions and rational beliefs, it seems to make sense to believe what we consciously experience.
Amazed by magic
This perceptual stubbornness is not just a feature of humans. Some primates have it too, as shown by their capacity to be amazed andamused by magic tricks. That is, they seem to understand that there’s a tension between what they’re seeing and what they know to be true. Given what we understand about their brains—specifically, that their perceptual neurons are also ‘recyclable’ for top-down functioning—the GAN theory suggests that these nonhuman animals probably have conscious experiences not dissimilar to ours.
The future of AI is more challenging. If we built a robot with a very complex GAN-style architecture, would it be conscious? On the basis of our theory, it would probably be capable of predictive coding, exercising the same machinery for perception as it deploys for top-down prediction or imagination. Perhaps like some current generative networks, it could “dream.” Like us, it probably couldn’t reason away its pain—and it might even be able to appreciate stage magic.
Theorizing about consciousness is notoriously hard, and we don’t yet know what it really consists in. So we wouldn’t be in a position to establish if our robot was truly conscious. Then again, we can’t do this with any certainty with respect to other animals either. At least by fleshing out some conjectures about the machinery of consciousness, we can begin to test them against our intuitions—and, more importantly, in experiments. What we do know is that a model of the mind involving an inner mechanism of doubt—a nit-picking system that’s constantly on the lookout for fakes and forgeries in perception—is one of the most promising ideas we’ve come up with so far.
Hakwan Lau is a professor of behavioral neuroscience at the University of California, Los Angeles, and he also holds an appointment at the University of Hong Kong. His work has been published in Science, Nature Neuroscience and Neuron among others.
This article was originally published at Aeon and has been republished under Creative Commons.
The post The Consciousness Battle appeared first on ORBITER.