Why I Became a Believer in Artificial Intelligence
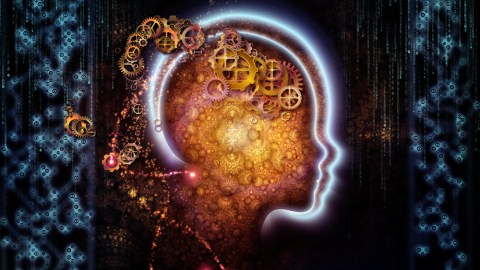
I’ve been asked periodically for a couple of decades whether I think artificial intelligence is possible. And I taught the artificial intelligence course at Columbia University. I’ve always been fascinated by the concept of intelligence. It’s a subjective word. I’ve always been very skeptical. And I am only now newly a believer.
Now this is subjective: my opinion is that IBM’s Watson computer is able to answer questions, and so, in my subjective view, that qualifies as intelligence. I spent six years in graduate school working on two things. One is machine learning and that’s the core to prediction – learning from data how to predict. That’s also known as predictive modeling. And the other is natural language processing or computational linguistics.
Working with human language really ties into the way we think and what we’re capable of doing and that does turn out to be extremely hard for computers to do. Now playing the TV quiz show Jeopardy means you’re answering questions – quiz show questions. The questions on that game show are really complex grammatically. And it turns out that in order to answer them Watson looks at huge amounts of text, for example, a snapshot of all the English speaking Wikipedia articles. And it has to process text not only to look at the question it’s trying to answer but to retrieve the answers themselves. Now at the core of this it turns out it’s using predictive modeling. Now it’s not predicting the future but it’s predicting the answer to the question.
The core technology is the same. In both cases it involves learning from examples. In the case of Watson playing the TV show Jeopardy it takes hundreds of thousands of previous Jeopardy questions from the TV show having gone on for decades and learns from them. And what it’s learning to do is predict whether this candidate answer to this question is likely to be the correct answer. So it’s going to come up with a whole bunch of candidate answers, hundreds of candidate answers, for the one question at hand at any given point in time. And then amongst all these candidate answers it’s going to score each one. How likely is it to be the right answer? And, of course, the one that gets the highest score as the highest vote of confidence – that’s ultimately the one answer it’s going to give.
So it’s not a yes/no thing. It’s trying to choose between a huge number of candidate answers and it has to choose the one correct answer to the question in order to be correct. And it is correct a great deal of the time. It knows how to assess its own confidence, buzz in on the game show if it feels it has a high confidence. And when it does, it is correct. It’s correct, I believe, about 90 or 92 percent of the time that it actually buzzes in to intentionally answer the question. The way it does this is by looking through all of this text to try to find all kinds of little evidence is this the right answer. And then once again, just like predicting a human’s behavior where you know a bunch of things about the person and you want to pull them all together – in this case you know a bunch of pieces of evidence.
Some of them are arcane, some of them are simplistic, some of them are grammatically deep but brittle, so there’s lots of errors – it’s not very trustable. But there’s a lot of them and you bring them all together with a predictive model that’s been derived automatically over the hundreds of thousands of learning examples from prior questions from prior TV episodes of this quiz show and by doing that, by driving it with all of that information, right. So it’s this sort of merging of many examples. Here’s a question and here’s what turned out to be the right answer and all the textual data. And it’s merging this together and creating – it emerges from that process the ability to sort of rattle off answers to questions.
You can go on YouTube and you can watch the episode where they aired the competition between IBM’s computer Watson and the all time two human champions of Jeopardy. And it just rattles off one answer after another. And it doesn’t matter how many years you’ve been looking at this. In fact, maybe the more years you’ve studied the ability or inability of computers to work with human language, the more impressive it is. It’s just rattling off one answer after another. I never thought that in my lifetime I would have cause to experience that the way I did which was, “Wow, that’s anthropomorphic. This computer seems like a person in that very specific skill set. That’s incredible. I’m gonna call that intelligent.”
Eric Siegel’s chapter detailing how IBM’s Watson works is in his new book, Predictive Analytics: The Power to Predict Who Will Click, Buy, Lie, or Die.
In Their Own Words is recorded in Big Think’s studio.
Image courtesy fo Shutterstock.