Moneyball on steroids: Can AI grant us 20/20 foresight?

- Moneyball popularized using big data in sports to inform decisions better than human intuition.
- AI’s ability to recognize patterns in vast amounts of data promises higher confidence when forecasting outcomes.
- Big Think Business spoke with the Probility AI team to discuss what leaders need to understand about this paradigm shift in decision-making.
A leader’s job is, first and foremost, to field a high-performing team. Your people must not only be able to execute their individual tasks well but also work together for the collective win. That requires careful consideration of each person’s skills, attitude, past experiences, future potential, and aptitude for cooperation.
Your tools for tackling this challenge are not dissimilar from the ones sports teams have used for decades. Scouts have their stats, and you have a stack of resumes. Scouts have their reports — for example, the 20-80 scale in baseball — and you have your evaluation criteria during interviews. And you both rely heavily on the “eye test,” in which your observations of a candidate’s actions and personality are filtered through your intuition to render a judgment.
The problem with these tools, especially the eye test, is that they come anchored to our cognitive biases. There are the usual suspects: age, gender, ethnicity, height, and so on. But even something as trifling as how someone sits in their chair or pronounces their W’s can tip our decisional scales — often in ways we aren’t consciously aware of. While we aren’t great at recognizing or accounting for these biases, we sure are good at rationalizing our decisions after the fact.
Things have shifted lately — for general managers of sports teams, at least. As popularized in the movie Moneyball (2011), analytics such as sabermetrics have used big data to cut through the subjective wisdom of the old guard and suss out the under-appreciated dimensions that predict player, and by extension team, success. Since then, sports and even other industries have had their “moneyball moments,” but the application has been uneven and success far from universal.
This short, and admittedly oversimplified, history will soon introduce a new chapter, one written by artificial intelligence.
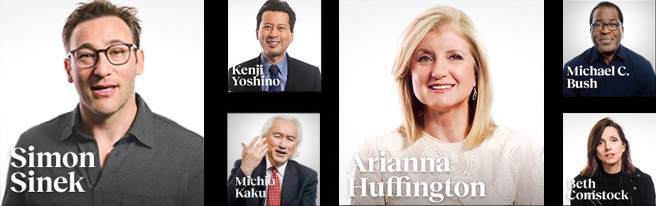
Probility AI, a sports data consultancy, is on a mission to harness the “deeper learning” of AI to make even better predictions about performance than Moneyball-esque methods. The company uses AI to help professional sports teams make informed decisions around risk management, training interventions, and personnel assessment. And while they focus on sports — for reasons we’ll get into later — this paradigm shift will likely have far-reaching consequences for leaders in many unique fields.
We sat down with Probility AI’s Doug Plocki (CEO), Nick Carone (CRO), and Quinton Krueger (board director) to discuss how AI can clarify the lessons of the past, why we must understand how these predictive machines work if we are to use them effectively, and why this shift has the potential to benefit anyone who relies on hindsight to inform future decisions.
Putting the past in focus
To consider how AI can bolster our decision-making toolkit — including the persuasive but often misleading effects of hindsight — Krueger asks us to imagine ourselves as the general manager of a sports team. (Feel free to pick the sport of your choice. It’s your team, after all.)
In this scenario, you’re trying to determine whether to re-sign a player who just turned 30. Your past experience suggests that this is the year bad things happen. Players become more prone to injury. Their performance begins to decline. Unable to keep up with the younger players, they slump into burnout and depression.
Given this, cutting ties seems sound, but hindsight also suggests some players buck these trends. Even if less capable than in their prime, they remain dependable and can act as mentors to the up-and-comers. To inform such a decision, you could turn to the power of an AI model such as Probility’s ARC engine — which stands for availability, reliability, and capability: shorthand for its measures of player potential.
ARC is trained on robust datasets and can analyze that data to suss out the historical patterns that help predict a player’s career capacity. It will evaluate, say, 20 years’ worth of data to determine things like how many games players played after turning 30, what their contributions were, how many injuries they suffered, and so on. It can then compare those to your 30-year-old player and the team’s current makeup.
Through AI, you’ve taken that handful of data points the human mind can reasonably manage and increased them exponentially. From that, Krueger notes, you’ll likely receive a report you don’t like, but if the confidence is high enough — and ARC can offer levels as high as 90% — you’ll need to take it seriously. That’s just the nature of being a leader.
“You might find you can get another 15 games out of this player’s career. That could be life-changing money for someone. Better yet, maybe you could prevent an injury by using AI to start to figure out [preventative] factors,” Krueger says.
He adds: “We’re answering old questions that have existed since the beginning of sports. We’re just using different tools and looking at the question with a different lens.”
Leaders need an AI game plan
With the current publicity surrounding AI, more models like the ARC engine are doubtless coming to many other fields and industries. To devise sound strategies, leaders must understand what these models can do and how they work.
Unfortunately, even in sports, most are dragging their feet. “One thing we’ve heard from GMs is, ‘Hey, that’s really cool stuff, but how do I use it?’” Carone says. “Part of the problem is that they just don’t understand what to do yet, and they have to learn.”
When we asked what leaders needed to know about utilizing AI, the Probility team warned that a model’s output depends greatly on the quality of its data. This is one reason they focused on sports. The field maintains a vast amount of vetted data that is both publicly available and fair use.
But all data collections aren’t created equal. Some industries won’t have robust enough historical data to make accurate predictions. Others may find their data sources are too fragmented for an AI to use properly. Finally, because data is created by humans, our biases can creep in. For instance, criminal risk assessment algorithms have displayed the same prejudices — when making sentencing and parole recommendations — that they were supposed to remove.
“That’s the only limitation on applying these types of tools: You’ve got to have data that support the model itself,” Plocki says. “In some cases, that’s just not going to be there, but in many it will.”
Any AI-led decision must make room for the intuition and lived-in knowledge that comes with years of experience.
Another potential pitfall would be if leaders either turned over their decision-making to an AI or ignored the technology altogether for fear they would be rendered obsolete. Both are mistakes because there will always be qualities of a person or situation that can’t be captured and quantified for an AI to use. These include people’s character, their cultural fit, their ability to support others, and, of course, random chance.
In fact, while Moneyball tells a riveting story, a reassessment of the Oakland A’s momentous season has suggested that the role of sabermetrics has been oversold. According to authors Sheldon and Alan Hirsch, the influence of starting pitchers like Barry Zito played no small part in the team’s success. Zito and players like him were well-scouted, hand-selected draft picks.
As such, any AI-led decision must make room for the intuition and lived-in knowledge that comes with years of experience. “It’s been part of our mindset from the beginning that we want to help people make better decisions, not replace them or make the decisions for them. So it’s always been our target to have the human at the center of what we’re doing,” Plocki says.
With an eye toward the future
The Probility team further notes that predictive AI wouldn’t just be useful for established organizations. That’s why the company made its founding mission to, as Kruger put it, “democratize AI and level the playing field for life-changing results.”
Consider, for instance, the Matthew principle. This is the tendency for those who already have money, success, or popularity to easily accrue more; meanwhile, those who don’t face a more difficult time. (Hence the name, which is an allusion to the Gospel of Matthew verse “For to everyone who has, more will be given.”)
The Matthew principle is what leads the wealthiest teams to woo star players, Silicon Valley giants to buy up the competition, and organizations with name recognition to build pipelines directly from Ivy League schools to their HR departments.
With AI assistance, teams or startups lacking deep reserves can still “scout at scale.”
With AI assistance, teams or startups lacking deep reserves can still “scout at scale.” They can research a larger swatch of potential team members and find the nontraditional or ignored star players that fit their particular team makeup best. They can then use those predictions to develop training and upskilling to help those players shine all the brighter.
AI can level the playing field for individual contributors, too. In sports, free agents could use AI to crunch the numbers and find the teams that match their skills best. They can then use that data to present their value during negotiations.
If we can find that balance between trusting our lived experiences and AI’s capability to manage and distill vast amounts of information, we can approach decisions with, if not 20/20 foresight, then more clarity than before. But that clarity only comes when we better understand the past we derive our decisions from.
“What we’ve learned is that GMs are looking at tomorrow or next week or next month,” Carone says. “They’re not looking back 20 years. They’re not even thinking about this at all, and they’re losing out on a lot of data that they could utilize to make decisions even today.”
Plocki adds, “You can use that to analyze and identify the methodologies, practices, etc., that are potentially useful and take that a step further as the tech evolves. That’s a good way to look at the sheer magnitude of information available about past successes and how that can be translated into future ones.”