The Neuroscience Power Crisis: What’s the fallout?
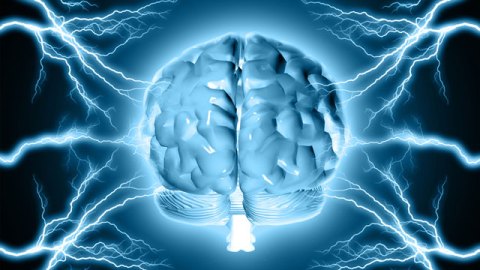
Last week a paper ($) was published in Nature Reviews Neuroscience that is rocking the world of neuroscience. The crack team of researchers including neuroscientists, psychologists, geneticists and statisticians analysed meta-analyses of neuroscience research to determine the statistical power of the papers contained within.
The group discovered that neuroscience as a field is tremendously underpowered, meaning that most experiments are too small to be likely to find the subtle effects being looked for and the effects that are found are far more likely to be false positives than previously thought. It is likely that many theories that were previously thought to be robust might be far weaker than previously imagined. This topic by its very nature is something that is very difficult to assess on the level of any individual study, but when the field is looked at as a whole, an assessment of the statistical power across a broad spread of the literature becomes possible and this has brought worrying implications.
Something that the research only briefly touches on is that neuroscience may not be alone. Underpowered research could indeed be endemic through other sciences besides neuroscience. This may be a consequence of institutionalised failings resulting in a spread of perverse incentives such as the pressure on scientists to churn out paper after paper rather than genuinely producing quality work. This has big implications on our assumption that science is self correcting; today in certain areas this may not necessarily be the case. I sat down with Katherine Button and Marcus Munafò, a couple of the lead researcherson the project, to discuss the impact of the research. The conversation is below:
I’d like to begin by asking you if any individual low powered studies you might have stumbled upon are particularly striking to you. I’m particularly curious of low powered studies that stand out as having made an impact on the field or perhaps ones that were the most heavily spun upon release or resulted in dubious interpretations.
K: We looked at meta-analyses and didn’t look directly at the individual studies which contributed to those meta-analyses. Some of the quality of the meta-analyses stood out because of unclear reporting of results; in some cases we had to work quite hard to extract the data, but because we were working at the meta level we weren’t really struck by the individual studies.
M: It’s probably worth taking a step back from this paper and thinking about the motivation for doing it in the first place, and the sort of things that gave rise to the motivation to write the paper. My research group is quite broad in its interests, so we do some genetic work, some human psychopharmacology work, I’ve worked with people on animal studies. Dating back several years, one of the consistent themes that was coming out of my research was that some effects that are apparently robust, if you read the published literature, are actually much harder to replicate than you might think. That’s true across a number of different fields; for example if you look at candidate gene studies, it is now quite widely agreed that most of these are just too small to detect an effect that would be plausible, given what we know about genetic effects now. A whole literature has built up around specific associations that captured the scientific imagination, but when you look at the data either through a meta-analysis, or by trying to replicate the finding yourself, you find it’s a lot more nebulous than some readings of the literature would have you believe. This was coming out as a really consistent theme. I started by doing meta-analysis as a way of identifying genetic variants robustly associated with outcomes so I could then genotype those outcomes myself, back in the day when genotyping was expensive. It proved that actually none of them was particularly robust, that was the clear finding.
I cut my teeth on meta-analytic techniques in that way and started applying the technique a bit more widely to human behavioural studies and so on, and one of the things that was really striking was that the average power in such diverse fields was really low – about 20%. That was the motivation behind looking at this more systematically and doing it in a way that would allow us to frame the problem, hopefully constructively, to an audience that might not have come across these problems in detail before. I could point at individual papers, but I’d be reluctant to, as that would say more about what I happen to have read rather than particularly problematic papers. It’s a broad problem, I don’t think it’s about a particular field or a particular method.
K: During my PhD I looked at emotional processing in anxiety and whether processing is biased towards a certain type of emotional expressions. In a naive reading of the literature, certain things came out, like there is a strong bias for fearful faces or disgusted faces, for example, but when I tried to replicate these findings, my results didn’t seem to fit. When I looked at the literature more critically, I realised that the reported effects were all over the place. I work in a medical department where there is an emphasis of the need for more reliable methods and statistical approaches, and Marcus was one of my PhD supervisors and had investigated the problems of low power in other literatures. Applying the knowledge gained from statistical methods training to critique the emotion processing literature lead me to think that a lot of this literature is probably false-positive. I wouldn’t be surprised if that was the same for other fields.
M: We tried to draw in people from a range of fields – John Ioannidis is an epidemiologist, Jonathan Flint is a psychiatric geneticist, Emma Robinson does animal model work and behavioural pharmacology, Brian Nosek is a psychologist, Kate works in a medical department, I work in a psychology department, and one of the points we try to make is that individual fields have learned some specific lessons. Clinical trials have learned about the value of pre-registration of study protocols and power analysis, genetics has learned about the importance of large scale consortial efforts, meta-analysis, stringent statistical criteria and replication. Many of those lessons could be brought together and applied more or less universally.
Can you explain the importance of meta-analyses for assessing the problem of underpowered research?
K: To work out the power that a study has to detect a true effect requires an estimation of the size of that true underlying effect. We can never really know what the true underlying effect is, so the best estimate we have is the effect size indicated by a meta-analysis, because that will be based on several studies’ attempt to measure that effect. We used the meta-analyses as a proxy for the true underlying effect and then went back and looked at the power the individual studies would have had assuming that meta-effect was actually true. That’s why you have to do this meta-analytic approach, because just calculating the power an individual study has to detect the effect observed in that study is circular and meaningless in this context.
M: We really are trying to be constructive – we don’t want this to be seen as a hatchet job. I think we’ve all made these kinds of mistakes in the past, certainly I have, and I’m sure I’ll continue to make mistakes without meaning to, but one of the advantages of this kind of project is that it’s made me think about how I can improve my own practices, such as by pre-registering study protocols.
K: And it’s not just mistakes, it’s also a practicality issue – resources are often limited. Yet even if you know your study is underpowered it’s still useful to say that “with this sample size, we can detect an effect of this is the size”. If you are upfront about the limitations of a small sample, then at least you know what size of effects you can and can’t detect, and interpret the results accordingly.
M: And make it clear when your study is confirmatory and when your study is exploratory – that distinction, I think, is blurred at the moment; my big concern is with the incentive structures that scientists have to work within. We are incentivised to crank the handle and run smaller studies that we can get published, rather than take longer to run fewer studies that might be more authoritative but aren’t going to make for as weighty a CV in the long run because, however much emphasis there is on quality, there is still an extent to which promotions and grant success are driven just by how heavy your CV is.
I’m also interested in how in your opinion neuroscience compares to psychology and other sciences more broadly in terms of the level of statistical power in published research, do you think neuroscience is an anomaly or is the problem equally prevalent across in other disciplines?
M: My sense is that wherever we’ve looked we’ve come up with the same answer. We haven’t looked everywhere but there is no field that has particularly stood out as better or worse, with the possible exception of phase three clinical trials that are funded by research councils without vested interests – those tend to be quite authoritative. But again, our motivation was not that neuroscience is particularly problematic – we were trying to raise these issues with a new audience and present some of the potential solutions that have been learned in fields such as genetics and clinical trials. It was more about reaching an audience than saying this field is better or worse than other fields because my sense is this is a universal problem.
Are there any particularly urgent areas you would like to highlight where under-powered research is an issue?
K: The emotional processing and anxiety literature – only because I am familiar with it. But I agree with Marcus’ point that these problems go across research areas and you are only familiar with them within the fields in which you work. I started off thinking that there were genuine effects to be found. There are so many studies with such conflicting evidence that you write a paper and try and say the evidence is conflicting and not very reliable, but then reviewers might say “how about so-and-so’s study?” and you just don’t have the space in papers to give a critique of all the methodological failings of all these studies.
M: I think there is a real distinction to be made between honest error where there are people who are trying to do a good job but they are incentivised to promote their findings and market their findings and it’s all unconscious and not malicious. There may be people who actually think of really gaming the system and don’t actually care whether or not they are right – that’s a really important distinction.
K: Something we do in my department is work with statisticians who are very careful about not overstating the claims of what we’ve found, I’ve done a few things looking at predictors of response to treatment which is effectively subgroup analysis of existing trial data and we try to be really upfront about the fact that these analyses are exploratory and that there are lots of limitations of subgroup analyses. I try to put at the forefront –‘type one and type two errors are possible and these findings need to be replicated before you believe any of them’. But as soon as you find a significant p-value, there are still a lot of reviewers that say ‘oh but this is really important for this, that or the other’ and no one wants to publish a nicely considered paper. There is a real emphasis from people saying ‘but why can’t you speculate on why this is really important and the implications this could have’ and you think that it could be important, but it could also be complete chance, so at every stage you are battling against the hyping up of your research.
M: I’ve had reviewers do this for us. In one case we were fairly transparent about presenting all our data and some of them were messy and some of them less so, and one of the reviewers said ‘just drop this stuff, it makes for a cleaner story and cleaner data if you don’t report all your data’ and we said ‘well actually we’d rather report everything and be transparent!’
K: As soon as you drop the nineteen things that didn’t come out, your one chance finding looks really amazing!
M: This is what I mean about honest error, the reviewer had no vested interest, the reviewer wasn’t trying to hype our results for us because – why would he or she? It’s just the system.
K: I think story telling is a real problem because a good story helps people to understand what your saying – it’s like when you write a blog you have to have a theme so people can follow you but there’s a balance to be struck between making your work accessible to readers but also not missing the point completely and going off on a tangent.
M: But that’s at the design stage; one of the things we are incentivised to do – wrongly in my opinion – is to include loads of measures so you’ve got a chance of finding something and then dropping all the other measures so it’s easier to tell the story. Actually what would be better is from the outset to design a study with relatively few outcomes where they all have their place and then you can write them up with all of them in there even if the results aren’t clear cut.
K: But that would require a lack of publication bias to really incentivise that, throwing all of your eggs into one basket is incentivised against really heavily. What we’ve tried to do recently when we are doing pilot studies, is writing in the protocols ‘we are going to be looking at all these different outcomes but this is our primary analysis and all these others are secondary exploratory analyses’. There are ways to report honestly and include lots of variables.
Q How big do you feel the gap is between bad science and institutionalised problems?
M: It’s not just about statistics; it takes a lot of guts as a PhD student to run the risk of having no publications at the end of your PhD.
K: It’s terrifying. Whether you get a post-doc depends on what your CV looks like.
M: I think of it as a continuum where there are very few people who are fraudulent, but then there are very few people who are perfect scientists, most of us are in the middle, where you become very invested in your ideas, there is confirmation bias, so one of the obvious things is you do an experiment as planned, you get exactly the results you expect and you think – great – and start writing it up, but if that process happens and you don’t get the results you were expecting you go back and check your data. So there can easily be a systematic difference in the amount of error checking that happens from one case to another, but in both cases there is the same likelihood that there will be errors in the data. It takes a lot of courage at the stage where you’ve run the analysis and got the results you were expecting to then go back and test them to destruction. Many scientists do this, but some don’t, not because they’re malicious but because that’s a natural psychological phenomenon – confirmation bias – you see what you are expecting to see.
Q Are there any specific bad practices that you think need to be highlighted?
M: Again, one of my main issues is with current incentive structures, which are hard for people to change from the bottom up – if you change your behaviour you are suddenly disadvantaged, relative to everyone else, in the short term. Then you have the problem that a lot of it is actually unconscious, well meant, non-malicious human instinct. Then you have the problem that when you do identify concerns there is no framework from which you say something without coming across as really hostile and confrontational – and that’s not necessarily constructive.
Many thanks to Katherine Button (@ButtonKate) and Marcus Munafò (@MarcusMunafo) fortaking part in this interview. You can keep up to date with their work by following their lab’s page on Twitter.
Reference:
Button KS, Ioannidis JP, Mokrysz C, Nosek BA, Flint J, Robinson ES, & Munafò MR (2013). Power failure: why small sample size undermines the reliability of neuroscience. Nature reviews Neuroscience, 14 (5), 365-76 PMID: 23571845
Image credit: Shutterstock/Feraru Nicolae