Can Science Be Trusted?
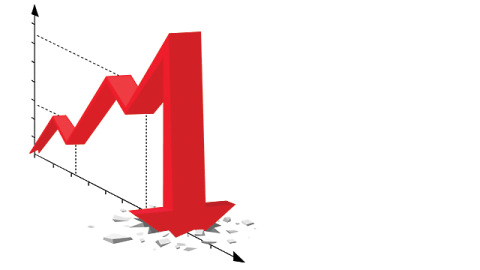
Can the scientific literature be trusted? In “Why Most Published Research Findings Are False,” Dr. John P. A. Ioannidis, Professor of Medicine and Director of the Stanford Prevention Research Center at Stanford University School of Medicine, basically says no, it cannot.
Far from a kook or an outsider, Dr. Ioannidis is considered one of the world’s foremost experts on the credibility of medical research. His work has been published in top journals (where it is heavily cited) and his efforts were favorably reviewed in a 2010 Atlantic article called “Lies, Damned Lies, and Medical Science.”
What kinds of analysis would allow Ioannidis to reach the conclusions he has reached? First know that a huge amount of work has been done in recent years to develop analytical methods for inferring publication bias by a variety of statistical methods. For example, there are now such accepted methodologies as Begg and Mazumdar’s rank correlation test, Egger’s regression, Orwin’s method, “Rosenthal’s file drawer,” and the now widely used “trim and fill” method of Duval and Tweedie. (Amazingly, at least four major software packages are available to aid detection of publication bias, for researchers doing meta-analyses. Read about it all here.)
There are many factors to consider when looking for publication bias. Take trial size. People who do meta-analysis of scientific literature have wanted, for some time, to have some reasonable way of compensating for the trial size of studies, because if you give small studies (which often have large variances in results) the same consideration as larger, more statistically significant studies, a handful of small studies with large effects sizes can unduly sway a meta-analysis. Aggravating this is the fact that studies showing a negative result are often rejected by journals or simply withheld from publication by their authors. When data goes unpublished, the literature that surfaces can give a distorted view of reality.
If you do a meta-analysis of a large enough number of studies and plot the effect size on the x-axis and standard error on the y-axis (giving rise to a “funnel graph”; see the graphic above, which is for studies involving Cognitive Behavioral Therapy), you expect to find a more-or-less symmetrical distribution of results around some average effect size, or failing that, at least a roughly equal number of data points on each side of the mean. For large studies, the standard error will tend to be small and data points will be high on the graph (because standard error, as usually plotted, goes from high values at the bottom of the y-axis to low numbers at the top; see illustration above). For small studies, the standard error tends (of course) to be large.
What meta-analysis experts have found is that quite often, the higher a study’s “standard error” (which is to say, the smaller the study), the more likely the study in question is to report a strongly positive result. So instead of a funnel graph with roughly equal data points on each side (which is what you expect statistically), you get a graph that’s visibly lopsided to the right, indicating that publication bias (from non-publication of “bad results”) is likely. Otherwise how do you account for the points mysteriously missing from the left side of the graph, in a graph that should (by statistical odds) have roughly equal numbers of points on both sides?
Small studies aren’t always the culprits. Some meta-analyses, in some research fields, show funnel-graph asymmetry at the top of the funnel as well as the bottom (in other words, across all study sizes). Data points are missing on the left side of the funnel. Which is hard to account for in a statistical distribution that should show points on both sides, in roughly equal amounts. The only realistic possibility is publication bias.
Then there’s the problem of spin-doctoring in studies that are published. This takes various forms, from changing the chosen outcomes-measure after all the data are in (to make the data look better, via a different criterion-of-success; one of many criticisms of the $35 million STAR-D study of depression treatments), “cherry-picking” trials or data points (which should probably be called pea-picking in honor of Gregor Mendel, who pioneered the technique), or the more insidious phenomenon of HARKing, Hypothesizing After the Results are Known, which often occurs with selective citation of concordant studies.
So is Dr. Ioannidis right? Are most published research findings false? I don’t think we have to go that far. I think it’s reasonable to say that most papers are probably showing real data, obtained legitimately. But we also have to admit there is a substantial phantom literature of unpublished data out there. (This is particularly true in pharmaceutical research, where it’s been shown that unflattering studies simply don’t get published.) And far too many study authors practice HARKing, cherry-picking, and post hoc outcome-measure swapping.
All of which is to say, it’s important to read scientific literature with a skeptical (or at least critical) eye. Fail to do that and you’re bound to be led astray, sooner or later.