MIT scientists design AI that could predict rare disasters, like bridge collapses and rogue waves
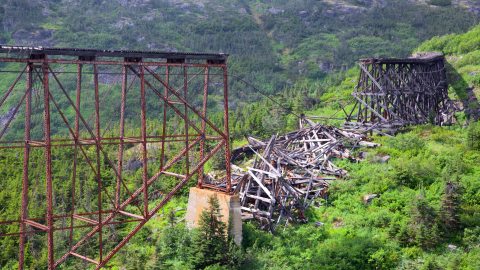
- Rare devastating events like massive earthquakes, pandemics, or rogue waves may seem random, but they might have telltale signs. We just don’t know how to find them.
- Artificial intelligence systems that use active learning can work to predict these events using very little data.
- This could be a useful tool for surviving on an occasionally unpredictable planet.
In 1995, the ocean liner Queen Elizabeth II was sailing off the coast of Newfoundland. The ship’s crew and passengers were caught in the teeth of a hurricane. The seas were a roiling mass, jostling the boat back and forth.
As his crew struggled to keep the boat afloat and the passengers huddled inside their cabins, Captain Ronald Warwick saw a wall of white rise before the boat. It seemed, he later recalled, as if the boat were heading straight for the White Cliffs of Dover. In horror, he realized this wall was not a landmass, but a wave dozens of feet high. A minute later, it smashed over the bow of his ship. The Queen Elizabeth II tipped forward and raced down the backside of the wave like a car on a roller coaster. It hit the next wave with enough force to damage the ship. Luckily, due to the fact the boat was not caught side-on, and most of the passengers were in their cabins, no one was injured.
The wave that hit the Queen Elizabeth II was more than twice as high as the waves that surrounded it. Such an event is dubbed a rogue wave — a large wave that seems to appear from nowhere.
Events like this — rare catastrophes that are truly damaging to people and property — are very difficult to predict. The category could include significant earthquakes, pandemics, or the unexpected failure of a bridge or a boat. Precisely because they are so rare, we have little data to predict when they may occur. Here, artificial intelligence might help by analyzing small data sets to extract the conditions that could lead to a rare event.
Making waves
Let’s take a deeper look at the example of the rogue wave. If we try to model wave height to predict when the next rogue wave might lift, we have a seemingly infinite number of variables to contend with: the distance between two successive waves, wave speed, the slope of the ocean floor, the presence of a storm nearby, or perhaps a butterfly flitting its wings in an African jungle.
Then there is the simple fact that rogue waves are rare. In fact, sailors who reported massive waves were long considered delusional. That disbelief was only broken on New Year’s Day of 1995, when a wave 84 feet tall hit the Draupner platform in the North Sea. The height of this wave was confirmed with a digital sensor, making it the first measured and recorded rogue wave.
Considering the lack of data and the list of variables involved in the generation of a rogue wave, predicting when and where they might occur seems just about impossible. Luckily, it is in situations like this that deep neural operators thrive.
AI can mine the smallest veins of data
Creating a purely mathematical model of earthquakes, waves, or pandemics is very difficult. In the real world, the underlying physics is complex, and it is hidden from us by a wall of noise. It is hard to pair a model based on pure physics with the true nature of what we see in the world around us.
Artificial intelligence, on the other hand, can allow us to model these systems without completely knowing the underlying equations. A team based out of Brown University and the Massachusetts Institute of Technology showed how we can pair AI with small data sets to predict rare and destructive events. Their results were recently published in Nature.
The team used a type of AI called active learning. “AI dynamically interacts with the underlying system of interest (i.e. rogue waves, hurricanes, pandemics, earthquakes) to acquire new data and efficiently learn the system,” Ethan Pickering, an expert in AI and the study’s lead author, told Big Think.
Even when using a small amount of data, which is all that is on offer for ultra-rare events, active learning can pick out what data is the most important, learning and adjusting each step of the way, and allowing it to be very efficient with small data sets. “This approach is sequential and allows the AI to update its understanding and decision-making ability with each new data point,” Pickering continues.
AI meets reality
Imagine a precursor to an enormous, devastating hurricane. You are sitting on a beautiful beach in the Caribbean with the perfect temperature and a gentle breeze. A nearby beach seems just as perfect. To understand how the AI finds a precursor to a hurricane, picture the AI analyzing the conditions at these two beaches. Starting from this small data set, it then builds a much larger data set, one that includes the conditions of many beaches. Advancing these through time would allow the AI to identify conditions that on any one beach look benign, but on a larger scale would lead to a massive hurricane.
“A precursor to a hurricane could be defined as the set of environmental conditions… ocean surface temperatures, water currents, air currents, and seemingly minor precipitation events,” Pickering explains.
These active learning systems can predict when and where rare events will happen. And, it even can predict events more extreme than any it has seen before (though there are limits to this — namely, a new set of data points may be required).
It is worth noting that AI is neither all-seeing nor capable of making sensible predictions on its own. Importantly, it cannot understand the system without the science that makes it work. As Pickering puts it, an “AI framework is a tool for scientists and researchers… not a replacement for fundamental science.” Oceanographers, biologists, geologists, climate modelers, or atmospheric scientists are still needed to feed the right information to the AI and guide it toward analyzing the parameters that truly can affect a system. For example, the timing between wave crests might affect the creation of a rogue wave, whereas other variables may not.
These active learning systems have many real-world applications, from forecasting pandemics and wildfires to structural failures — a useful tool for surviving on an occasionally unpredictable planet.