How cell phone data can help redesign cities
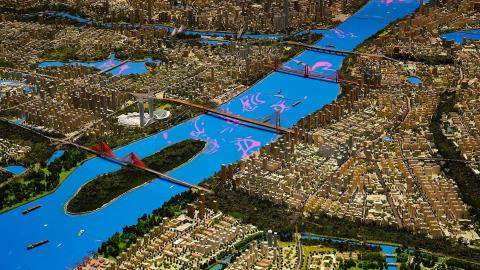
Credit: Getty Images
- A treasure trove of mobility data from devices like smartphones has allowed the field of “city science” to blossom.
- I recently was part of team that compared mobility patterns in Brazilian and American cities.
- We found that, in many cities, low-income and high-income residents rarely travel to the same geographic locations. Such segregation has major implications for urban design.
Almost 55 percent of the world’s seven billion people live in cities. And unless the COVID-19 pandemic puts a serious — and I do mean serious — dent in long-term trends, the urban fraction will climb almost to 70 percent by midcentury. Given that our project of civilization is staring down a climate crisis, the massive population shift to urban areas is something that could really use some “sciencing.”
Is urbanization going to make things worse? Will it make things better? Will it lead to more human thriving or more grinding poverty and inequality? These questions need answers, and a science of cities, if there was such a thing, could provide answers.
Good news. There already is one!
The science of cities
With the rise of Big Data (for better or worse), scientists from a range of disciplines are getting an unprecedented view into the beating heart of cities and their dynamics. Of course, really smart people have been studying cities scientifically for a long time. But Big Data methods have accelerated what’s possible to warp speed. As “exhibit A” for the rise of a new era of city science, let me introduce you to the field of “human mobility” and a new study just published by a team I was on.
Human mobility is a field that’s been amped up by all those location-enabled devices we carry around and the large-scale datasets of our activities, such as credit card purchases, taxi rides, and mobile phone usage. These days, all of us are leaving digital breadcrumbs of our everyday activities, particularly our movements around towns and cities. Using anonymized versions of these datasets (no names please), scientists can look for patterns in how large collections of people engage in daily travel and how these movements correlate with key social factors like income, health, and education.
There have been many studies like this in the recent past. For example, researchers looking at mobility patterns in Louisville, Kentucky found that low-income residents tended to travel further on average than affluent ones. Another study found that mobility patterns across different socioeconomic classes exhibit very similar characteristics in Boston and Singapore. And an analysis of mobility in Bogota, Colombia found that the most mobile population was neither the poorest nor the wealthiest citizens but the upper-middle class.
These were all excellent studies, but it was hard to make general conclusions from them. They seemed to point in different directions. The team I was part of wanted to get a broader, comparative view of human mobility and income. Through a partnership with Google, we were able to compare data from two countries — Brazil and the United States — of relatively equal populations but at different points on the “development spectrum.” By comparing mobility patterns both within and between the two countries, we hoped to gain a better understanding of how people at different income levels moved around each day.
Mobility in Brazil vs. United States
The results were remarkable. In a figure from our paper (shown above), it’s clear that we found two distinct kinds of relationship between income and mobility in cities.
The first was a relatively sharp distinction between where people in lower and higher income brackets traveled each day. For example, in my hometown of Rochester, New York or Detroit, the places visited by the two income groups (e.g., job sites, shopping centers, doctors’ offices) were relatively partitioned. In other words, people from low-income and high-income neighborhoods were not mixing very much, meaning they weren’t spending time in the same geographical locations. In addition, lower income groups traveled to the city center more often, while upper income groups traveled around the outer suburbs.
The second kind of relationship was exemplified by cities like Boston and Atlanta, which didn’t show this kind of partitioning. There was a much higher degree of mixing in terms of travel each day, indicating that income was less of a factor for determining where people lived or traveled.
In Brazil, however, all the cities showed the kind of income-based segregation seen in U.S. cities like Rochester and Detroit. There was a clear separation of regions visited with practically no overlap. And unlike the U.S., visits by the wealthy were strongly concentrated in the city centers, while the poor largely traversed the periphery.
Data-driven urban design
Our results have straightforward implications for city design. As we wrote in the paper, “To the extent that it is undesirable to have cities with residents whose ability to navigate and access resources is dependent on their socioeconomic status, public policy measures to mitigate this phenomenon are the need of the hour.” That means we need better housing and public transportation policies.
But while our study shows there are clear links between income disparity and mobility patterns, it also shows something else important. As an astrophysicist who spent decades applying quantitative methods to stars and planets, I am amazed at how deep we can now dive into understanding cities using similar methods. We have truly entered a new era in the study of cities and all human systems. Hopefully, we’ll use this new power for good.