Why free will is required for true artificial intelligence
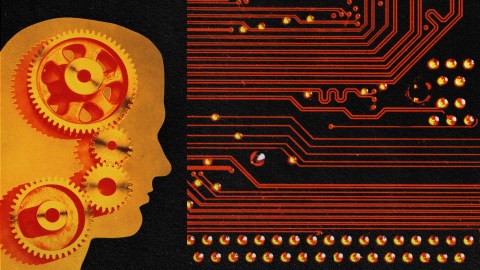
- The most sophisticated of generative AI systems can have trouble with novel scenarios not represented in the training data.
- While reaching superhuman performance in many areas, AI has not achieved the same success in things that most humans — and animals — find easy.
- Artificial general intelligence (AGI) may have to be earned through the exercise of agency.
The field of artificial intelligence (AI) has always taken inspiration from neuroscience, starting with the field’s founding papers, which suggested that neurons can be thought of as performing logical operations. Taking a cue from that perspective, most of the initial efforts to develop AI focused on tasks requiring abstract, logical reasoning, especially in testing grounds like playing chess or Go, for example — the kinds of things that are hard for most humans. The successes of the field in these arenas are well known.
Recent years have witnessed stunning advances in other areas like image recognition, text prediction, speech recognition, and language translation. These were achieved mainly due to the development and application of deep learning, inspired by the massively parallel, multilevel architecture of the cerebral cortex. This approach is tailor-made for learning the statistical regularities in masses and masses of training data. The trained neural networks can then abstract higher-order patterns; for example, recognizing types of objects in images. Or they can predict what patterns will be most likely in new instances of similar data, as in the autocompletion of text messages or the prediction of the three-dimensional structures of proteins.
When trained in the right way, the neural networks can also generate wholly new examples of types of data they have seen before. Generative models can be used, for example, to create “a realistic photo image of a horse on the top of Mt. Everest” or a “picture of an ice cream van in the style of van Gogh.” And “large language models” can produce what look like very reasonable and cogent passages of text or responses to questions. Indeed, they are capable of having conversations that give a strong impression that they truly understand what they are being asked and what they are saying — to the point where some users even attribute sentience to these systems.
However, even the most sophisticated systems can quickly be flummoxed by the right kind of questioning, the kind that presents novel scenarios not represented in the training data that humans can handle quite easily. Thus, if these systems have any kind of “understanding” — based on the abstraction of statistical patterns in an unimaginably vast set of training data — it does not seem to be the kind that humans have.
Indeed, while reaching superhuman performance in many areas, AI has not achieved the same success in things that most humans find easy: moving around in the world, understanding causal relations, or knowing what to do when faced with a novel situation. Notably, these are things that most animals are good at too: they have to be to survive in challenging and dynamic environments.
These limitations reflect the fact that current AI systems are highly specialized: They’re trained to do specific tasks on the basis of the patterns in the data they encountered. But when asked to generalize, they often fail, in ways that suggest they did not, in fact, abstract any knowledge of the underlying causal principles at play. They may “know” that when they see X, it is often followed by Y, but they may not know why that is: whether it reflects a true causal pattern or merely a statistical regularity, like night following day. They can thus make predictions for familiar types of data but often cannot translate that ability to other types or to novel situations.
Thus, the quest for artificial general intelligence has not made the same kind of progress as AI systems aimed at particular tasks. It is precisely that ability to generalize that we recognize as characteristic of natural intelligence. The mark of intelligence in animals is the ability to act appropriately in novel and uncertain environments by applying knowledge and understanding gained from past experience to predict the future, including the outcomes of their own possible actions. Natural intelligence thus manifests in intelligent behavior, which is necessarily defined normatively as good or bad, relative to an agent’s goals. To paraphrase Forrest Gump, intelligent is as intelligent does.
The other key aspect of natural intelligence is that it is achieved with limited resources. That includes the computational hardware, the energy involved in running it, the amount of experience required to learn useful knowledge, and the time it takes to assess a novel situation and decide what to do. Greater intelligence is the ability not just to arrive at an appropriate solution to a problem but to do so efficiently and quickly. Living organisms do not have the luxury of training on millions of data points, or running a system taking megawatts of power, or spending long periods of time exhaustively computing what to do. It may in fact be precisely those real-world pressures that drive the need and, hence, the ability to abstract general causal principles from limited experience.
Current AI systems are highly specialized: They’re trained to do specific tasks on the basis of the patterns in the data they encountered.
Understanding causality can’t come from passive observation, because the relevant counterfactuals often do not arise. If X is followed by Y, no matter how regularly, the only way to really know that is a causal relation is to intervene in the system: to prevent X and see if Y still happens. The hypothesis has to be tested. Causal knowledge thus comes from causal intervention in the world. What we see as intelligent behavior is the payoff for that hard work.
The implication is that artificial general intelligence will not arise in systems that only passively receive data. They need to be able to act back on the world and see how those data change in response. Such systems may thus have to be embodied in some way: either in physical robotics or in software entities that can act in simulated environments.
Artificial general intelligence may have to be earned through the exercise of agency.