Can we stop AI hallucinations? And do we even want to?
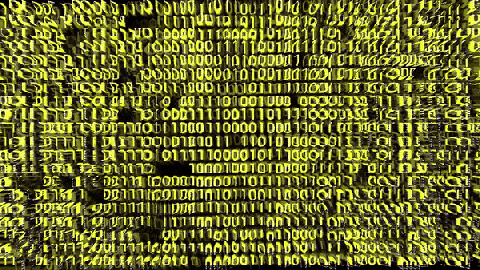
- “Hallucinations” occur when an AI, such as a large language model, generates mistaken or made-up information as fact.
- AIs hallucinate because the algorithms generate responses based on statistical probability, not reasoning or understanding.
- Making AIs more deterministic may reduce the prevalence of hallucinations, but at the expense of more creative output.
As AI continues to advance, one major problem has emerged: “hallucinations.” These are outputs generated by the AI that have no basis in reality. Hallucinations can be anything from small mistakes to downright bizarre and made-up information. The issue makes many people wonder whether they can trust AI systems. If an AI can generate inaccurate or even totally fabricated claims, and make it sound just as plausible as accurate information, how can we rely on it for critical tasks?
Researchers are exploring various approaches to tackle the challenge of hallucinations, including using large datasets of verified information to train AI systems to distinguish between fact and fiction. But some experts argue that eliminating the chance of hallucinations entirely would also require stifling the creativity that makes AI so valuable.
The stakes are high, as AI is playing an increasingly important role in sectors from healthcare to finance to media. The success of this quest could have far-reaching implications for the future of AI and its applications in our daily lives.
Why AI hallucinates
Generative AI systems like ChatGPT sometimes produce “hallucinations” — outputs that are not based on real facts — because of how these systems create text. When generating a response, the AI essentially predicts the likely next word based on the words that came before it. (It’s a lot more sophisticated than how your phone keyboard suggests the next word, but it’s built on the same principles.) It keeps doing this, word by word, to build complete sentences and paragraphs.
The problem is that the probability of some words following others is not a reliable way to ensure that the resulting sentence is factual, Chris Callison-Burch, a computer and information science professor at the University of Pennsylvania, tells Freethink. The AI might string together words that sound plausible but are not accurate.
“As soon as you make the model more deterministic … you will destroy the quality.”
Maria Sukhareva
ChatGPT’s struggle with basic math highlights the limitations of its text generation approach. For instance, when asked to add two numbers it had encountered in its training data, like “two plus two,” it could correctly answer “four.” However, this was because it assigned a high probability to the word “four” following the phrase “two plus two equals,” not because it understood the mathematical concepts of numbers and addition.
This example shows how the system’s reliance on patterns in its training data can lead to failures in tasks that require genuine reasoning, even in simple arithmetic.
“But if you took two very long numbers that it had never seen before, it would simply generate an arbitrary lottery number,” Callison-Burch said. “This illustrates that this kind of auto-regressive generation that is used by ChatGPT and similar language learning models (LLMs) makes it difficult to perform kinds of fact-based or symbolic reasoning.”
Casual AI
Eliminating hallucinations is a tough challenge because they are a natural part of how a chatbot works. In fact, the varying, slightly random nature of its text generation is part of what makes the quality of these new AI chatbots so good.
“As soon as you make the model more deterministic, basically you force it to predict the most likely word, you greatly restrict hallucinations, but you will destroy the quality as the model will always generate the same text,” Maria Sukhareva, an AI expert at Siemens said in an interview.
While eliminating hallucinations using LLMs is likely not possible, effective techniques have been developed to reduce their prevalence, noted Callison-Burch. One promising approach is called “retrieval augmented generation.” Instead of relying only on the AI’s existing training data and the context provided by the user, the system can search for relevant information on Wikipedia or other web pages. It then uses this (presumably more factual) information to generate more accurate summaries or responses.
Another approach to reducing hallucination is to use “causal AI,” which allows the AI to test different scenarios by altering variables and examining the problem from multiple perspectives.
“Getting the data set right and establishing guardrails for what is considered to be reasonable outcomes can prevent the hallucinations from ever coming to light,” Tony Fernandes, the founder of UserExperience.ai, told Freethink. “However, the ultimate answer is that no matter how sophisticated the AI process is, humans will need to stay involved and provide oversight.”
Liran Hason, who leads Aporia, a company that helps reduce AI mistakes, says that to stop AI from making things up, we should learn from how the cyber security world made firewalls to stop hackers’ data intrusions. The key lies in implementing AI guardrails — proactive measures designed to filter and correct AI outputs in real-time. These guardrails act as a first line of defense, identifying and rectifying hallucinations and thwarting potential malicious attacks.
“Completely eliminating hallucinations is challenging because these AI apps rely on knowledge sources that might contain inaccuracies or outdated information,” he added.
Impact on creativity
When it comes to AI-generated content, hallucinations can be a double-edged sword. In situations where accuracy is crucial, such as diagnosing medical conditions, offering financial advice, or summarizing news events, these deviations from reality can be problematic and even harmful, Callison-Burch said.
However, in the realm of creative pursuits like writing, art, or poetry, AI hallucinations can be a valuable tool. The fact that they stray from existing factual information and venture into the imagination can fuel the creative process, allowing for novel and innovative ideas to emerge.
“For instance, if I may want to mimic my own creative writing style, I can retrieve examples of past stories that I’ve written and then have the LLM follow along in a similar style,” he added.
“When it comes to AI models, we can have it both ways.”
Kjell Carlsson
A link between hallucinations and creativity in AI systems parallels what we see in human imagination. Just like people often come up with creative ideas by letting their minds wander beyond the boundaries of reality, AI models that generate the most innovative and original outputs also tend to be more prone to occasionally producing content that isn’t grounded in real-world facts, Kjell Carlsson, head of AI Strategy at Domino Data Lab, noted in an interview.
“There are obviously times for AI models and people when this is more than justified in order to prevent harm,” he added. “However, when it comes to AI models, we can have it both ways. We can and should eliminate hallucinations at the level of a given AI application because — for it to be adopted and deliver impact — it must behave as intended as much as possible. However, we can also remove these constraints, provide less context, and use these AI models to promote our own creative thinking.”
This article was originally published by our sister site, Freethink.