Predictive Analytics: “Freakonomics” Meets Big Data
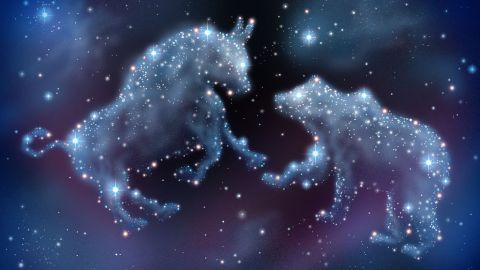
While writing my book, Predictive Analytics: The Power to Predict Who Will Click, Buy, Lie, or Die, a certain well-known book strongly influenced me to make the science accessible, relevant, and even entertaining: Freakonomics: A Rogue Economist Explores the Hidden Side of Everything.
Both Freakonomics and Predictive Analytics are more than just books—they are disciplines of insight, schools of analytical thought. So, who would win in a fight—Freakonomics or Predictive Analytics?
Freakonomics Versus Predictive Analytics
Champion: Freakonomics, the entertaining pop science originator of what I would call “humanized econometrics.” This book takes the analytical, data driven approach to economics—know by some as econometrics—and applies it to everyday life, including real estate brokers, drug dealers, and nursery schools. It defuses the sometimes dry perception of economics with a healthy dose of “Freak” in the book’s title.
Challenger: Predictive Analytics, the accessible—yet conceptually complete—primer to, well, predictive analytics. This book defuses the sometimes intimidating perception of data driven prediction and even defines the field of study itself with its semi-humorous subtitle, “The Power to Predict Who Will Click, Buy, Lie, or Die.”
Reviewers of my book have drawn the parallel. Founding Advertising.com executive Stein Kretsinger dubbed it, “The Freakonomics of big data,” KDnuggets Editor Gregory Piatetsky-Shapiro said it “does to Analytics what Freakonomics did to Economics,” and icrunchdata cofounder Todd Nevis declared it to be, “Outfreakingstanding” (Todd wasn’t actually drawing a comparison to Freakonomics, but I thought I’d mention that anyway).
How do the two compare? The field of predictive analytics has one major thing in common with—and one major difference from—Freakonomics.
The Commonality: Freaky Data Discoveries Are Predictive
As with the anecdotally rich discoveries in Freakonomics, practitioners of predictive analytics constantly stumble upon insightful gems such as, vegetarians miss fewer flights.
Welcome to the “Ripley’s Believe It or Not!” of data science. Predictive analytics’ aim isn’t only to assess human hunches by testing relationships that seem to make sense, but also to explore a boundless playing field of possible truths beyond the realms of intuition. And so, at times, the analysis drops onto one’s desk connections that seem to defy logic. As strange, mystifying, or unexpected as they may seem, these discoveries help predict.
Here are a ten such discoveries from my book, Predictive Analytics
Insights—Far Out and Freaky, Yet Predictive:
1. Insight: Banner ads affect you more than you think. Although you may feel you’ve learned to ignore them, people who see a merchant’s banner ad are 61 percent more likely to subsequently perform a related search, and this drives a 249 percent increase in clicks on the merchant’s paid textual ads in the search results.
Company: Yahoo!
Suggested Explanation: Advertising exerts a subconscious effect.
2. Insight:Friends stick to the same cell phone company (a social effect). If you switch wireless carriers, your contacts are in turn up to seven times more likely to follow suit.
Company: a major North American wireless carrier
Suggested Explanation: People experience social influence and/or heed financial incentives for in-network calling.
Company: Orbitz
Suggested Explanation: Macs are often more expensive than Windows computers, so Mac users may on average have greater financial resources.
4. Insight:Your e-mail address reveals your level of commitment. Customers who register for a free account with an Earthlink.com e-mail address are almost five times more likely to convert to a paid, premium-level membership than those with a Hotmail.com e-mail address.
Company: an online dating website
Suggested Explanation: Disclosing permanent or primary e-mail accounts reveals a longer-term intention.
6. Insight:Guys literally drool over sports cars. Male college student subjects produce measurably more saliva when presented with images of sports cars or money.
Company: Northwestern University Kellogg School of Management
Suggested Explanation: Consumer impulses are physiological cousins of hunger.
7. Insight:Low credit rating, more car accidents. If your credit score is higher, car insurance companies will lower your premium, since you are a lower driving risk. People with poor credit ratings are charged more for car insurance. In fact, a low credit score can increase your premium more than an at-fault car accident; missing two payments can as much as double your premium.
Company:automobile insurers
Suggested Explanation: “Research indicates that people who manage their personal finances responsibly tend to manage other important aspects of their life with that same level of responsibility, and that would include being responsible behind the wheel of their car,” Donald Hanson of the National Association of Independent Insurers theorizes.
8. Insight:Retirement is bad for your health. For a certain working category of males in Austria, each additional year of early retirement decreases life expectancy by 1.8 months.
Company: University of Zurich
Suggested Explanation: Unhealthy habits such as smoking and drinking follow retirement. Voltaire said, “Work spares us from three evils: boredom, vice, and need,” and Malcolm Forbes said, “Retirement kills more people than hard work ever did.”
9. Insight:Smokers suffer less from repetitive motion disorder. In certain work environments, people who smoke cigarettes are less likely to develop carpal tunnel syndrome.
Company:a major metropolitan newspaper, conducting research on its own staff’s health
Suggested Explanation: Smokers take more breaks.
10. Insight:Solo rockers die younger than those in bands. Although all rock stars face higher risk, solo rock stars suffer twice the risk of early death as rock band members.
Company:public health offices in the UK
Suggested Explanation: Band members benefit from peer support and solo artists exhibit even riskier behavior.
The Difference: Predictive Analytics Drives Operational Decisions
While these eye-catching observations are right up Freakonomics’ alley, they serve a different purpose for predictive analytics. Instead of enlightening us and unveiling insights as an end in and of itself, they are just the beginning for prediction. These kinds of discoveries—plus many others that may be less entertaining yet are equally powerful—form the building blocks for a predictive model.
Freakonomics’ objective is to elucidate. One of its coauthors calls the other an “intellectual detective” who, per their book’s subtitle, “Explores the hidden side of everything.” Definitely cool. But predictive analytics’ objective is a entirely different.
More than just striving to elucidate, predictive analytics runs the world. It actively drives operational decisions central to organizations’ functions in order to improve the very way in which companies, government agencies, healthcare facilities, and law enforcement serve and treat us. The technology’s prediction of human behavior determines, millions of times a day, whom to call, mail, approve, test, diagnose, warn, investigate, incarcerate, set up on a date, and medicate. By answering this mountain of questions, predictive analytics combats financial risk, fortifies healthcare, conquers spam, toughens crime-fighting, boosts sales—and may in fact answer the biggest question of all: How can we improve the effectiveness of all these massive functions across business and government sectors?
To this end, predictive analytics techniques focus narrowly, striving to discover from data only insights that serve for the particular predictive objective at hand, such as whether each individual will consume, work, love, procreate, vote, mess up, commit a crime, or even die. The underlying analytical learning process builds on individual insights such as those in the table above to form a predictive model, a mechanism (such as a formula or set of business rules) that can consider in concert the multitude of factors known about an individual in order to form its “best bet” prediction for that individual. These predictive capabilities are developed by following a number-crunching, trial-and-error process that has its roots in statistics and computer science.
Of course, the elucidating insights from Freakonomics, economics, and all social sciences for that matter, do inform decision making. But it’s not the same thing. Social science insights drive a smaller number of strategic decisions, usually in an ad hoc manner in which decision makers may or may not consider such insights in doing their jobs. In contrast, predictive analytics drives a large number of operational and tactical decisions in a systematic or automatic fashion. Social sciences set out to inform we humans; predictive analytics sets out systematically improve organizational functions on a massive scale.
What’s So Big About Big Data?
This endeavor to apply prediction addresses a burning question: With all the excitement over “big data”—which has recently witnessed such dramatic hype as to assume the status of a Movement—what is the value, the function, the purpose? Answer: The most actionable thing to be gained from data is prediction. This is achieved by analytically learning from data how to render predictions for each individual. In this way, predictive analytics is the means to tap big data’s full potential.
Big data grows wildly, organically. In this heyday of big data, we thus make hay of this valuable overgrowth of “weeds,” the residual side effect of organizations conducting business as usual. This is in contrast to economics and other social sciences, which often must actively conduct experimental studies in order to accrue pertinent data.
Isn’t Prediction Impossible?
Freakonomics coauthor Stephen Dubner is skeptical. “The first step toward predicting the future is admitting you can’t,” he once said (“Why Can’t We Predict Earthquakes?” Freakonomics Radio, 2011). As the Danish physicist Niels Bohr put it, “Prediction is very difficult, especially if it’s about the future.” People are unpredictable. Better data helps prediction more than better math and models do, but we’ll never predict with high accuracy.
Fortunately, we don’t need to. The massive operations of companies and government agencies benefit greatly by playing the odds better, tipping the numbers games they already play in our favor. Simply predicting a nice amount better than guessing does the trick; a hazy view of what’s to come outperforms complete darkness by a landslide. I call this The Prediction Effect: A little prediction goes a long way.
I Never Meta-Science I Didn’t Like
Although illuminating insights often emerge as a secondary effect, predictive analytics strives not so much to understand how the world works as to actively improve how it works. This makes predictive analytics a kind of “metascience” that transcends the taxonomy of natural and social sciences, abstracting across them by learning from any and all data sources that would typically serve biology, criminology, economics (and Freakonomics), education, epidemiology, medicine, political science, psychology, or sociology. Predictive analytics’ mission is to engineer solutions. As for the data employed and the insights gained, the tactic in play is, “whatever works.”
More about Predictive Analytics:
Eric Siegel, Ph.D., is the founder of Predictive Analytics World (www.pawcon.com)—coming in 2013 and 2014 to Toronto, San Francisco, Chicago, Washington D.C., Boston, Berlin, and London—and the author of Predictive Analytics: The Power to Predict Who Will Click, Buy, Lie, or Die (February 2013, published by Wiley). Interested in employing predictive analytics at your organization? Get started with the Predictive Analytics Guide (www.pawcon.com/guide).